String and Array Functions in SQL for Data Science
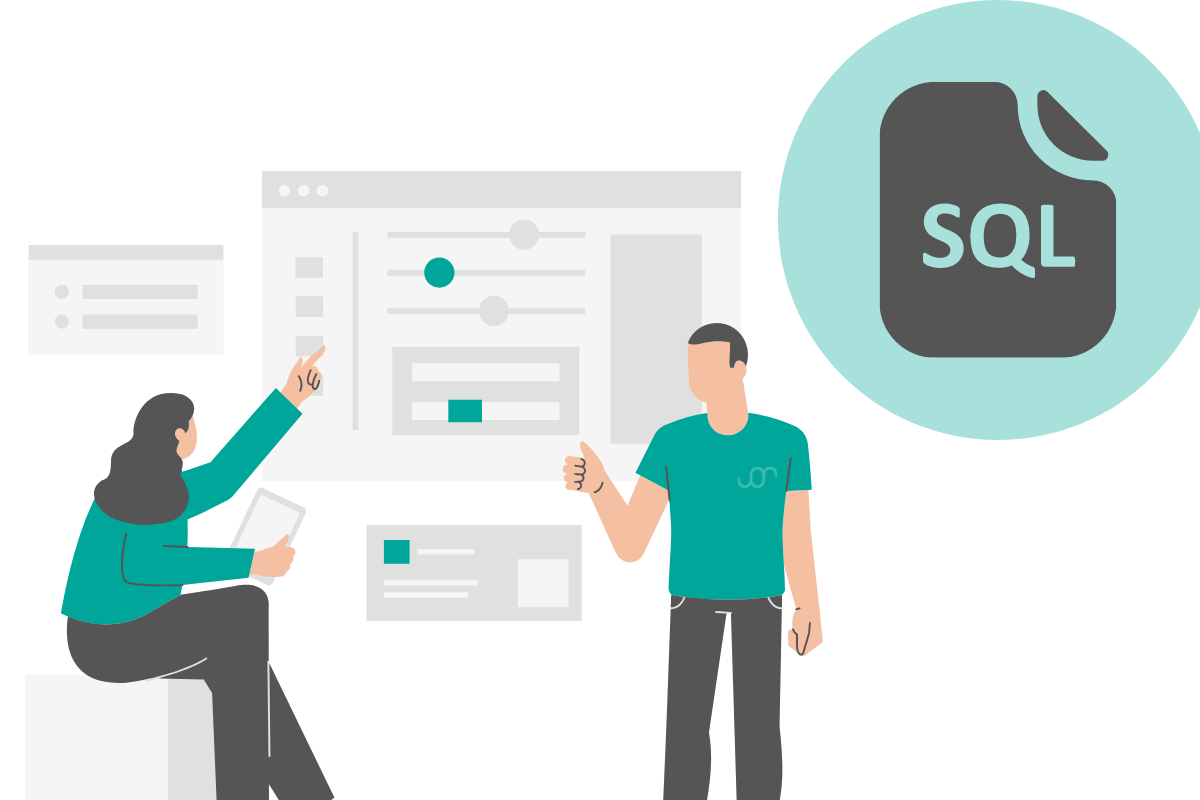
Categories
Commonly used string and array functions in SQL Data Science Interviews.
Commonly used string and array functions in SQL Data Science Interviews.
In a previous article "SQL Scenario Based Interview Questions", we had touched upon the various date and time functions in SQL. In this article, we will look at another important favorite topic in Data Science Interviews – string and array manipulation. With increasingly diverse and unstructured data sources becoming commonplace, string and array manipulation has become a integral part of Data Analysis and Data Science functions. The key ideas discussed in this article include
- Cleaning Strings
- String Matching
- String Splitting
- Creating Arrays
- Splitting Arrays to rows
- Aggregating text fields
You might also want to look at our Pandas article on string manipulation in DataFrame as we use quite a few similar concepts here as well.
String Matching
Let us start with a simple string-matching problem. This is from a past City of San Fransisco Data Science Interview Question.
Find the number of violations that each school had
Determine the number of violations for each school. Any inspection that does not have risk category as null is considered a violation. Print the school’s name along with the number of violations. Order the output in the descending order of the number of violations.

You can solve this problem here: https://platform.stratascratch.com/coding/9727-find-the-number-of-violations-that-each-school-had
The problem uses the sf_restaurant_health_violations dataset with the following fields.
sf_restaurant_health_violations
business_id | int |
business_name | varchar |
business_address | varchar |
business_city | varchar |
business_state | varchar |
business_postal_code | float |
business_latitude | float |
business_longitude | float |
business_location | varchar |
business_phone_number | float |
inspection_id | varchar |
inspection_date | datetime |
inspection_score | float |
inspection_type | varchar |
violation_id | varchar |
violation_description | varchar |
risk_category | varchar |
The relevant data in the table looks like this.
business_id | business_name | business_address | business_city | business_state | business_postal_code | business_latitude | business_longitude | business_location | business_phone_number | inspection_id | inspection_date | inspection_score | inspection_type | violation_id | violation_description | risk_category |
---|---|---|---|---|---|---|---|---|---|---|---|---|---|---|---|---|
5800 | John Chin Elementary School | 350 Broadway St | San Francisco | CA | 94133 | 37.8 | -122.4 | {'longitude': '-122.403154', 'needs_recoding': False, 'latitude': '37.798358', 'human_address': '{"address":"","city":"","state":"","zip":""}'} | 5800_20171017 | 2017-10-17 | 98 | Routine - Unscheduled | 5800_20171017_103149 | Wiping cloths not clean or properly stored or inadequate sanitizer | Low Risk | |
64236 | Sutter Pub and Restaurant | 700 Sutter St | San Francisco | CA | 94102 | 37.79 | -122.41 | {'longitude': '-122.41188', 'needs_recoding': False, 'latitude': '37.78881', 'human_address': '{"address":"","city":"","state":"","zip":""}'} | 64236_20170725 | 2017-07-25 | 88 | Routine - Unscheduled | 64236_20170725_103133 | Foods not protected from contamination | Moderate Risk | |
1991 | SRI THAI CUISINE | 4621 LINCOLN Way | San Francisco | CA | 94122 | 37.76 | -122.51 | {'longitude': '-122.507779', 'needs_recoding': False, 'latitude': '37.764073', 'human_address': '{"address":"","city":"","state":"","zip":""}'} | 1991_20171129 | 2017-11-29 | 86 | Routine - Unscheduled | 1991_20171129_103139 | Improper food storage | Low Risk | |
3816 | Washington Bakery & Restaurant | 733 Washington St | San Francisco | CA | 94108 | 37.8 | -122.41 | {'longitude': '-122.405845', 'needs_recoding': False, 'latitude': '37.795174', 'human_address': '{"address":"","city":"","state":"","zip":""}'} | 3816_20160728 | 2016-07-28 | 67 | Routine - Unscheduled | 3816_20160728_103108 | Contaminated or adulterated food | High Risk | |
39119 | Brothers Restaurant | 4128 GEARY Blvd | San Francisco | CA | 94118 | 37.78 | -122.46 | {'longitude': '-122.463762', 'needs_recoding': False, 'latitude': '37.781148', 'human_address': '{"address":"","city":"","state":"","zip":""}'} | 39119_20160718 | 2016-07-18 | 79 | Routine - Unscheduled | 39119_20160718_103133 | Foods not protected from contamination | Moderate Risk |
The relevant columns are business_name and risk_category
These columns are populated thus.
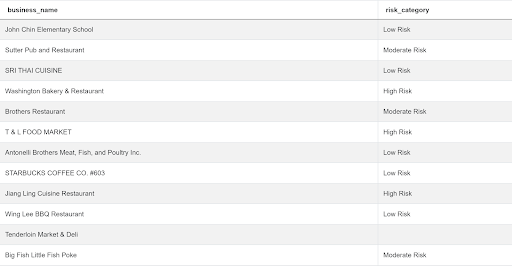
Approach and Solution
This is a relatively straightforward problem. We need
- Identify “Schools” from the business category
- Count the violations excluding the rows where the risk_category is NULL
The simplest string-matching function in SQL is the LIKE function that searches for a substring inside a larger string. However, one needs to use wildcards to ensure the correct match is found. Since we do not know for sure that Schools end with the word School, we use the % wildcard before and after the string to ensure that the word “SCHOOL” is searched for. Further, we use the ILIKE function to make a case-insensitive search. The solution is now very simple.
SELECT business_name,
COUNT(*) AS num_violations
FROM sf_restaurant_health_violations
WHERE business_name ILIKE '%SCHOOL%'
AND risk_category IS NOT NULL
GROUP BY 1 ;
If your SQL flavor does not have the ILIKE statement, we can convert the string to upper or lower case and then use the LIKE statement.
Splitting a Delimited String
Now that we have warmed up with string search, let us try another common string manipulation technique: splitting. There are numerous use cases for splitting a string. Splitting a string requires a delimiter (a separator). To illustrate this let us look at a problem from another City of San Francisco Data Science Interview problem.
Business Density Per Street
Find the highest and the average number of businesses in all the streets. Only consider those streets that have five or more businesses. Assume that the second word in the Address field represents the street name for the business. Note there might be multiple entries for the same business in the dataset. Count each business only once.
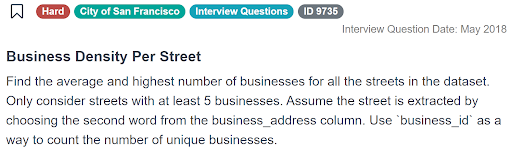
You can solve the problem on the StrataScratch Platform here: https://platform.stratascratch.com/coding/9735-business-density-per-street
This problem uses the same sf_restaurant_health_violations used in the previous problem. The fields of interest for this problem are business_id and business_address which are populated thus.
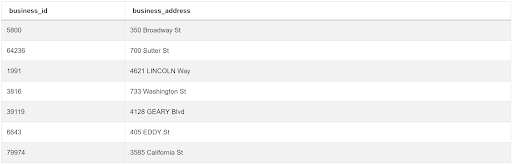
Approach and Solution
We need to extract the second word from the address. That represents the street name. To do this we split the string using space as a delimiter (separator) and extracting the second word. We can do this using the SPLIT_PART function. This is similar to the split() method in Python. Since Postgres is case sensitive, we convert the output to upper case.
SELECT UPPER(split_part(business_address, ' ', 2)) AS streetname,
business_address
FROM sf_restaurant_health_violations ;
We get the following output.
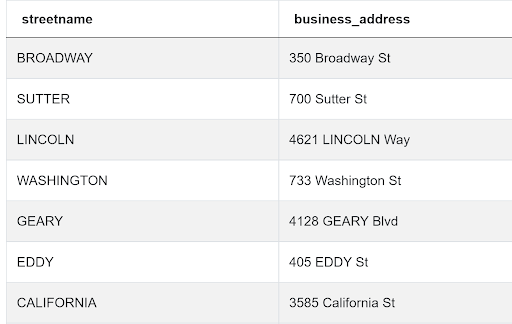
Now the problem becomes relatively easy to solve. We find the number of distinct business entries on each street. Since we need only those businesses with five or more entries, we use the HAVING clause to subset the output.
SELECT UPPER(split_part(business_address, ' ', 2)) AS streetname,
COUNT (DISTINCT business_id) AS density
FROM sf_restaurant_health_violations
GROUP BY 1
HAVING COUNT (DISTINCT business_id) >= 5 ;
We get the following output.

Now we can aggregate this table using a subquery, CTE or a temp table. We have used a CTE in this case and get the final output.
WITH rel_businesses AS
(SELECT UPPER(split_part(business_address, ' ', 2)) AS streetname,
COUNT (DISTINCT business_id) AS density
FROM sf_restaurant_health_violations
GROUP BY 1
HAVING COUNT (DISTINCT business_id) >= 5)
SELECT AVG(density),
MAX(density)
FROM rel_businesses ;
Arrays
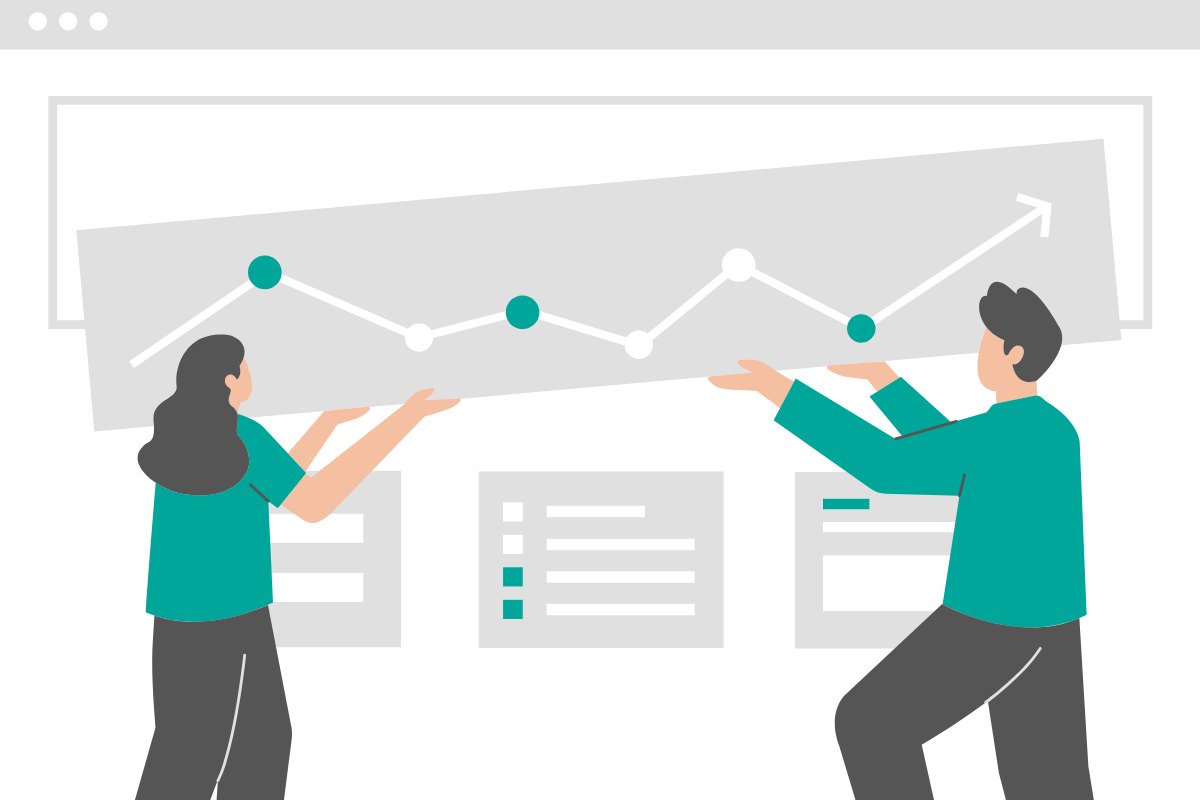
Most modern SQL flavors allow creation and manipulation of arrays. Let us look at working with string arrays. One can manipulate integer and floating-point arrays in a similar manner. To illustrate this let us take an SQL Data Science Interview problem for an AirBnB interview.
City With Most Amenities
Find the city with most amenities in the given dataset. Each row in the dataset represents a unique host. Output the name of the city with the most amenities.
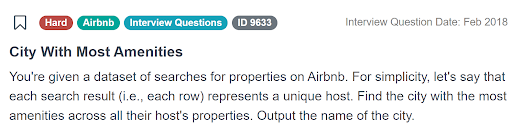
You can solve the problem here: https://platform.stratascratch.com/coding/9633-city-with-most-amenities
The problem uses the airbnb_search_details dataset with the following fields.
airbnb_search_details
id | int |
price | float |
property_type | varchar |
room_type | varchar |
amenities | varchar |
accommodates | int |
bathrooms | int |
bed_type | varchar |
cancellation_policy | varchar |
cleaning_fee | bool |
city | varchar |
host_identity_verified | varchar |
host_response_rate | varchar |
host_since | datetime |
neighbourhood | varchar |
number_of_reviews | int |
review_scores_rating | float |
zipcode | int |
bedrooms | int |
beds | int |
id | price | property_type | room_type | amenities | accommodates | bathrooms | bed_type | cancellation_policy | cleaning_fee | city | host_identity_verified | host_response_rate | host_since | neighbourhood | number_of_reviews | review_scores_rating | zipcode | bedrooms | beds |
---|---|---|---|---|---|---|---|---|---|---|---|---|---|---|---|---|---|---|---|
12513361 | 555.68 | Apartment | Entire home/apt | {TV,"Wireless Internet","Air conditioning","Smoke detector","Carbon monoxide detector",Essentials,"Lock on bedroom door",Hangers,Iron} | 2 | 1 | Real Bed | flexible | FALSE | NYC | t | 89% | 2015-11-18 | East Harlem | 3 | 87 | 10029 | 0 | 1 |
7196412 | 366.36 | Cabin | Private room | {"Wireless Internet",Kitchen,Washer,Dryer,"Smoke detector","First aid kit","Fire extinguisher",Essentials,"Hair dryer","translation missing: en.hosting_amenity_49","translation missing: en.hosting_amenity_50"} | 2 | 3 | Real Bed | moderate | FALSE | LA | f | 100% | 2016-09-10 | Valley Glen | 14 | 91 | 91606 | 1 | 1 |
16333776 | 482.83 | House | Private room | {TV,"Cable TV",Internet,"Wireless Internet",Kitchen,"Free parking on premises","Pets live on this property",Dog(s),"Indoor fireplace","Buzzer/wireless intercom",Heating,Washer,Dryer,"Smoke detector","Carbon monoxide detector","First aid kit","Safety card","Fire extinguisher",Essentials,Shampoo,"24-hour check-in",Hangers,"Hair dryer",Iron,"Laptop friendly workspace","translation missing: en.hosting_amenity_49","translation missing: en.hosting_amenity_50","Self Check-In",Lockbox} | 2 | 1 | Real Bed | strict | TRUE | SF | t | 100% | 2013-12-26 | Richmond District | 117 | 96 | 94118 | 1 | 1 |
1786412 | 448.86 | Apartment | Private room | {"Wireless Internet","Air conditioning",Kitchen,Heating,"Suitable for events","Smoke detector","Carbon monoxide detector","First aid kit","Fire extinguisher",Essentials,Shampoo,"Lock on bedroom door",Hangers,"translation missing: en.hosting_amenity_49","translation missing: en.hosting_amenity_50"} | 2 | 1 | Real Bed | strict | TRUE | NYC | t | 93% | 2010-05-11 | Williamsburg | 8 | 86 | 11211 | 1 | 1 |
14575777 | 506.89 | Villa | Private room | {TV,Internet,"Wireless Internet","Air conditioning",Kitchen,"Free parking on premises",Essentials,Shampoo,"translation missing: en.hosting_amenity_49","translation missing: en.hosting_amenity_50"} | 6 | 2 | Real Bed | strict | TRUE | LA | t | 70% | 2015-10-22 | 2 | 100 | 90703 | 3 | 3 |
The main fields of interest here are city and amenities that are populated thus.
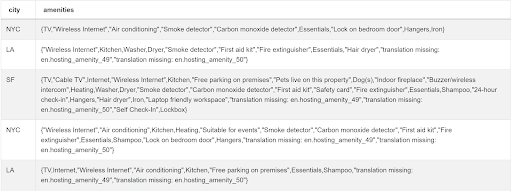
Approach and solution
To solve this let us break this problem into parts.
- We need to find the number of amenities for a given property
- Aggregate the amenities at city level
- Find the city with the highest number of amenities.
The amenities are represented in form of a string separated by commas. However, SQL right now recognizes this field as string. So, we need to convert this string into individual amenities by splitting them using the comma delimiter. To do this we use the STRING_TO_ARRAY() function and specify comma as the delimiter.
SELECT city,
STRING_TO_ARRAY(amenities, ',') AS num_amenities
FROM airbnb_search_details ;
We get the following output.
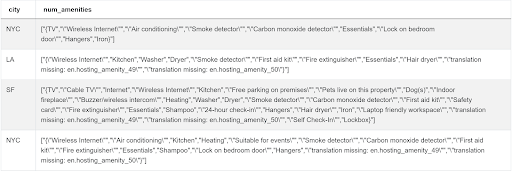
Note for this problem, opening and closing braces are considered a part of the first and last word in the string. If we want to eliminate to clean the string, we can use the BTRIM function. BTRIM function will remove all the leading and trailing characters specified. We can modify our query in the following manner.
SELECT city,
STRING_TO_ARRAY(BTRIM(amenities, '{}'), ',') AS num_amenities
FROM airbnb_search_details ;
This gives us the following output. As one can see we have successfully removed the leading and trailing braces.
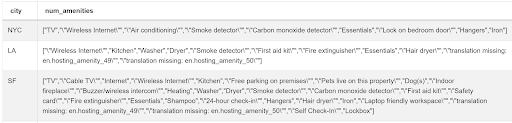
To find the number of amenities, we need to count the number of elements in the amenities array. We can do this by using the ARRAY_LENGTH() function. The function requires us to specify the array dimension whose length is to be specified. This is useful for multi-dimensional arrays. Since our array is 1-dimensional, we simply specify 1.
SELECT city,
ARRAY_LENGTH(STRING_TO_ARRAY(BTRIM(amenities, '{}') , ',') , 1) AS num_amenities
FROM airbnb_search_details ;
Our output looks like this
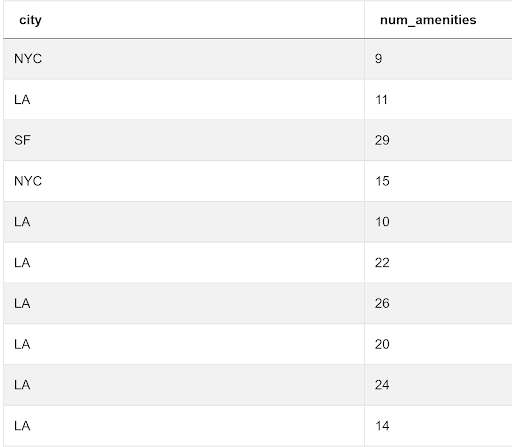
We now proceed to aggregate the number of amenities at city level.
SELECT city,
SUM(ARRAY_LENGTH(STRING_TO_ARRAY(BTRIM(amenities, '{}'), ','), 1)) AS num_amenities
FROM airbnb_search_details
GROUP BY 1 ;
Our output now looks like this.
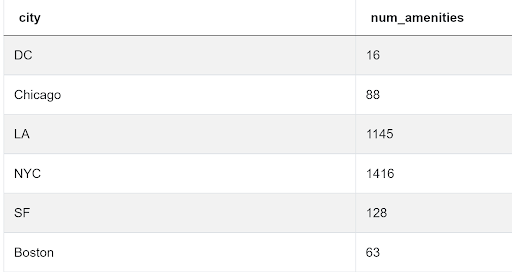
We can now find the city with the highest number of amenities by sorting in descending order and using LIMIT 1 or more reliably, by ranking them.
SELECT CITY
FROM
(SELECT city,
DENSE_RANK() OVER (
ORDER BY num_amenities DESC) AS rank
FROM
(SELECT city ,
SUM(ARRAY_LENGTH(STRING_TO_ARRAY(BTRIM(amenities, '{}'), ','), 1)) AS num_amenities
FROM airbnb_search_details
GROUP BY 1) Q1) Q2
WHERE rank = 1 ;
Splitting an Array
The above problem could have also been solved by exploding the array into individual rows and then aggregating the number of amenities for each city. Let us use this method in another SQL data science question from Meta (Facebook) interview.
Views Per Keyword
Find the number of views for each keyword. Report the keyword and the total views in the decreasing order of the views.
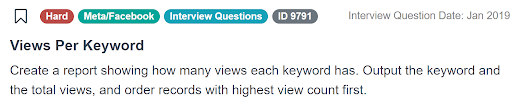
You can solve the problem here: https://platform.stratascratch.com/coding/9791-views-per-keyword
The problem uses the facebook_posts and facebook_post_views datasets. The fields present in the facebook_posts dataset are
facebook_posts
post_id | int |
poster | int |
post_text | varchar |
post_keywords | varchar |
post_date | datetime |
The data is presented in the following manner
post_id | poster | post_text | post_keywords | post_date |
---|---|---|---|---|
0 | 2 | The Lakers game from last night was great. | [basketball,lakers,nba] | 2019-01-01 |
1 | 1 | Lebron James is top class. | [basketball,lebron_james,nba] | 2019-01-02 |
2 | 2 | Asparagus tastes OK. | [asparagus,food] | 2019-01-01 |
3 | 1 | Spaghetti is an Italian food. | [spaghetti,food] | 2019-01-02 |
4 | 3 | User 3 is not sharing interests | [#spam#] | 2019-01-01 |
The facebook_post_views has the following fields
facebook_post_views
post_id | int |
viewer_id | int |
And this is how the data in looks
post_id | viewer_id |
---|---|
4 | 0 |
4 | 1 |
4 | 2 |
5 | 0 |
5 | 1 |
Approach and Solution
Let us break this problem into individual parts.
- We start off by merging the two datasets on the post_id field. We need to aggregate the number of views for each post
SELECT fp.post_id,
fp.post_keywords,
COALESCE(COUNT(DISTINCT fpv.viewer_id), 0) AS num_views
FROM facebook_posts fp
LEFT JOIN facebook_post_views fpv ON fp.post_id = fpv.post_id
GROUP BY 1,
2 ;
We get the following output.
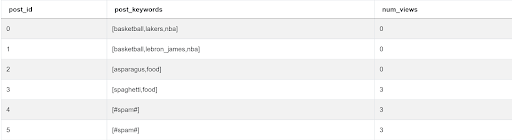
- We need to assign the views to each keyword. For example for post_id = 3, the keyword sphagetti and the keyword food should each get 3 views. For post_id = 4, the spam keyword should get 3 views and so on. To accomplish this, we first clean the string stripping the brackets and the # symbol.
SELECT fp.post_id,
STRING_TO_ARRAY(BTRIM(fp.post_keywords, '[]#'), ',') AS keyword,
COALESCE(COUNT(DISTINCT fpv.viewer_id), 0) AS num_views
FROM facebook_posts fp
LEFT JOIN facebook_post_views fpv ON fp.post_id = fpv.post_id
GROUP BY 1,
2 ;
We get the following output
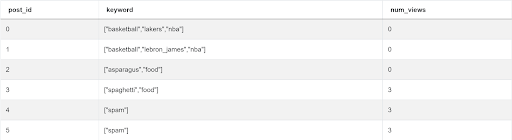
- Now we separate (explode) the array into individual records using the UNNEST function.
SELECT fp.post_id,
UNNEST(STRING_TO_ARRAY(BTRIM(fp.post_keywords, '[]#'), ',')) AS keyword,
COALESCE(COUNT(DISTINCT fpv.viewer_id), 0) AS num_views
FROM facebook_posts fp
LEFT JOIN facebook_post_views fpv ON fp.post_id = fpv.post_id
GROUP BY 1,
2 ;
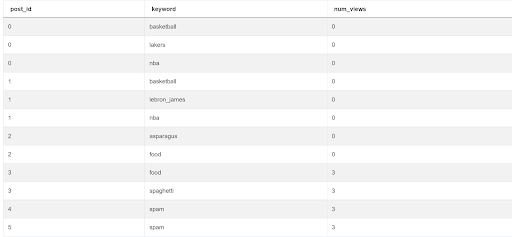
- We can now easily aggregate the number of views per keyword and sort them in descending order.
WITH exp_keywords AS
(SELECT fp.post_id ,
UNNEST(STRING_TO_ARRAY(BTRIM(fp.post_keywords, '[]#'), ',')) AS keyword ,
COALESCE(COUNT(DISTINCT fpv.viewer_id), 0) AS num_views
FROM facebook_posts fp
LEFT JOIN facebook_post_views fpv ON fp.post_id = fpv.post_id
GROUP BY 1,
2)
SELECT keyword,
sum(num_views) AS total_views
FROM exp_keywords
GROUP BY 1
ORDER BY 2 DESC ;
Aggregating Text Fields
Let us finish things off by doing the converse. Aggregating rows back into a string. We illustrate this with a SQL Data Science Interview question from Google.
File Contents Shuffle
Rearrange the words of the filename final.txt to make a new file named wacky.txt. Sort all the words in alphabetical order, output the words in column and the filename wacky.txt in another.
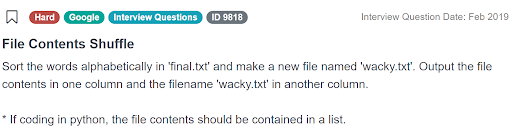
You can solve the problem here: https://platform.stratascratch.com/coding/9818-file-contents-shuffle
The problem uses the google_file_store dataset with the following columns.
google_file_store
filename | varchar |
contents | varchar |
The contents of the dataset look like this.
filename | contents |
---|---|
draft1.txt | The stock exchange predicts a bull market which would make many investors happy. |
draft2.txt | The stock exchange predicts a bull market which would make many investors happy, but analysts warn of possibility of too much optimism and that in fact we are awaiting a bear market. |
final.txt | The stock exchange predicts a bull market which would make many investors happy, but analysts warn of possibility of too much optimism and that in fact we are awaiting a bear market. As always predicting the future market is an uncertain game and all investors should follow their instincts and best practices. |
Approach and Solution
Let us solve this problem in a step-wise manner.
- We first keep only the contents of the filename final.txt, split the contents using space as a delimiter, explode the resulting array into individual rows and sort in alphabetical order.
SELECT UNNEST(STRING_TO_ARRAY(CONTENTS, ' ')) AS words
FROM google_file_store
WHERE filename ILIKE '%FINAL%'
ORDER BY 1 ;
We get the following output
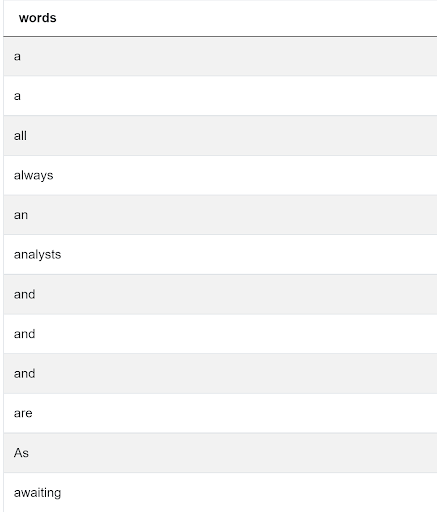
- We now need to combine the individual words back into a string. To do this we use the STRING_AGG() function and specify space as the delimiter. This function is similar to the join() method in Python. We also add a filename for the new string and output.
WITH exploded_arr AS
(SELECT UNNEST(STRING_TO_ARRAY(CONTENTS, ' ')) AS words
FROM google_file_store
WHERE filename ILIKE '%FINAL%'
ORDER BY 1)
SELECT 'wacky.txt' AS filename,
STRING_AGG(words, ' ') AS CONTENTS
FROM exploded_arr ;
Conclusion
In this article we looked at the text and array manipulation abilities of SQL. This is specifically useful in ETL process upstream as well as Analysis downstream. As with other Data Science areas, only patience, persistence and practice can make you proficient. On StrataScratch, we have over 700 coding and non-coding problems that are relevant to Data Science Interviews. These problems appeared in actual Data Science interviews at top companies like Google, Amazon, Microsoft, Netflix, et al. For e.g., check out our posts "40+ Data Science Interview Questions From Top Companies" and "The Ultimate Guide to SQL Interview Questions" to practice such interview questions and prepare for the most in-demand jobs at big tech firms and start-ups across the world.
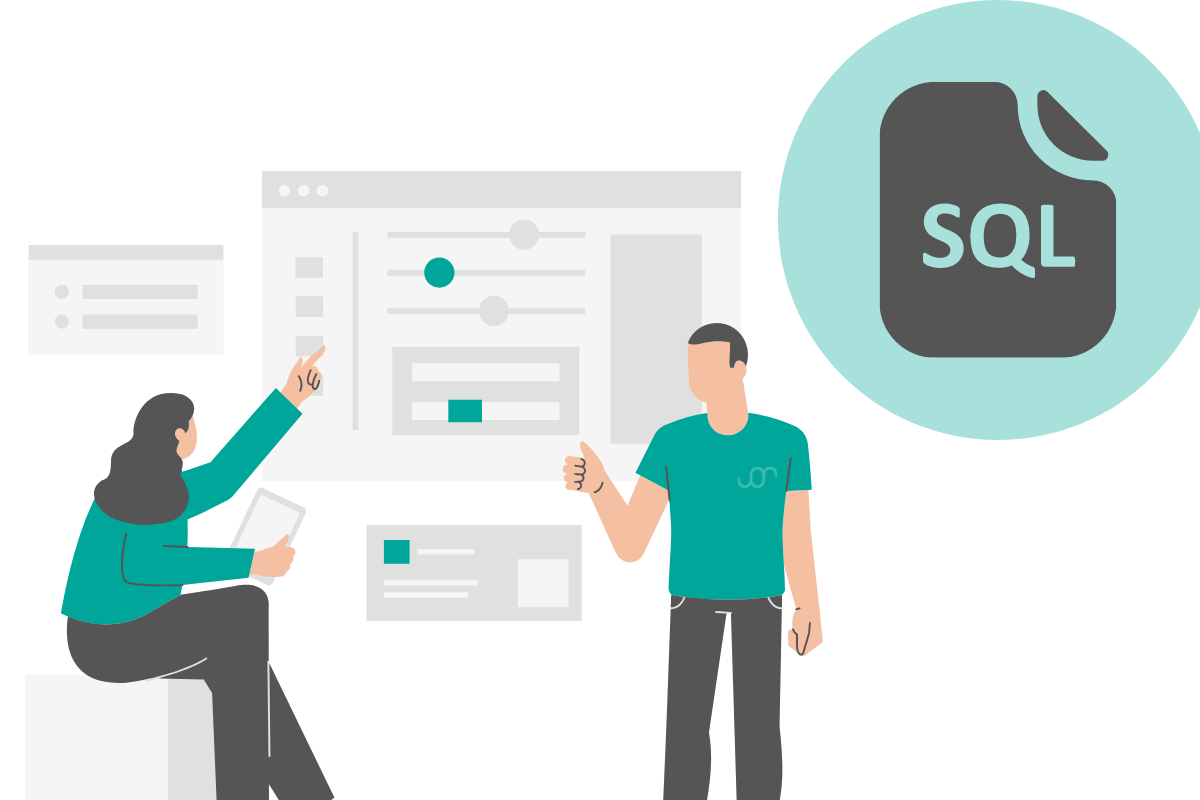