How FAANG companies are leveraging data science and AI
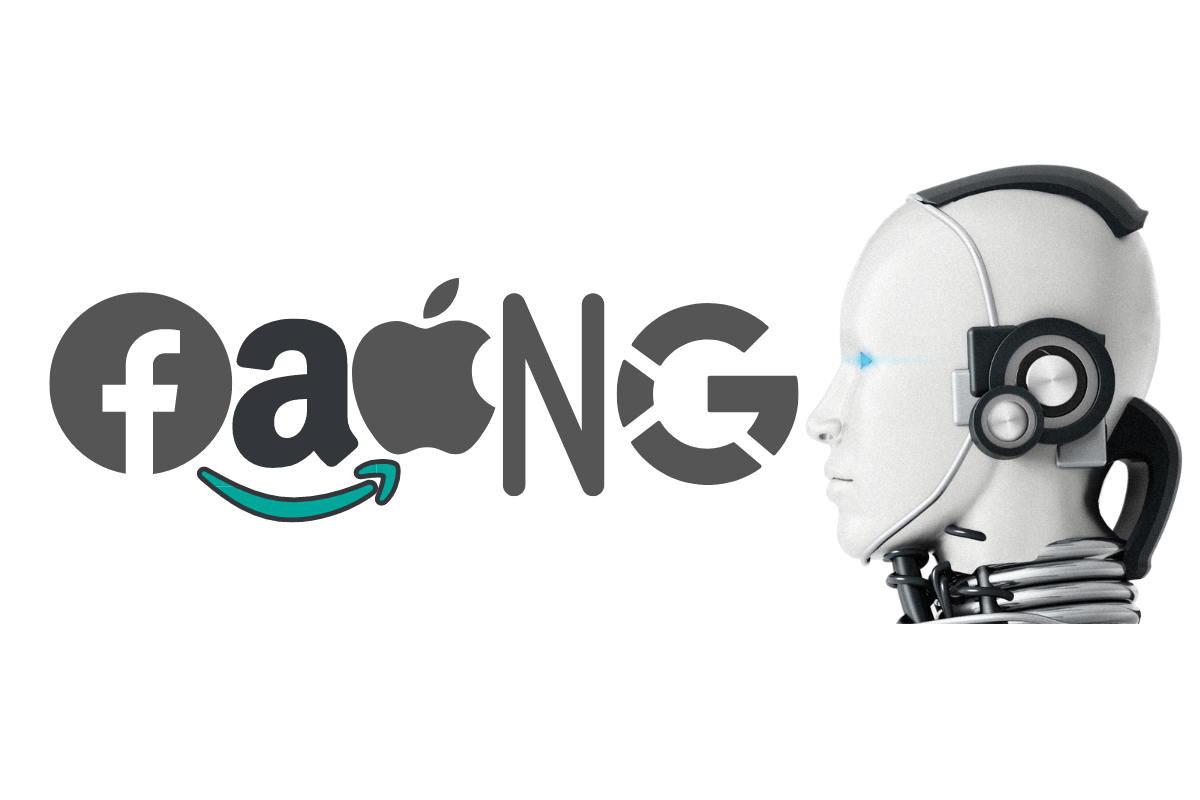
- Written by:
Nathan Rosidi
This article will cover information about how FAANG companies leverage Data Science and AI to drive product innovation and thereby improve their customer satisfaction and drive revenue growth.
As the data is increasing at an exponential rate, most of the companies are leveraging this data to drive growth and enhance customer experience. Big Data has spread around the world since the 1960s. Data that contains greater variety, arriving in growing columns, and with increased velocity is what Oracle defines as big data. Every industry has seen a rise in the number of data science firms that analyze this data for commercial insights. There are many data science companies that have improved over time due to data-driven decision making. In this article, we will be talking about FAANG companies. FAANG stands for Facebook/Meta, Amazon, Apple, Netflix and Google.
Big Data is useless without the knowledge of experts who can transform cutting-edge technology into useful insights. The value of a data scientist who knows how to wring relevant insights out of gigabytes of data is rising as more and more firms today unlock the power of big data.
Data processing and analysis have a huge value, and it is becoming increasingly obvious as time goes on. The importance a data scientist holds in a company is still largely unknown to executives, despite the fact that they are aware of how data science is a hot business and how data scientists are like modern-day superheroes.
Why do companies need Data Science Capabilities?
In order to succeed in this ever-changing world, companies need to rely heavily on data driven decisions and make their products more innovative. Some examples of innovative products backed by data science include Alexa by Amazon which is a virtual assistant and does basic jobs using voice commands, Google’s apps like translate, maps, etc., Netflix's recommendation system to show users what they might like, etc. Having such innovative products backed by data helps the companies in building a great customer experience which improves customer loyalty and thereby drives growth.
Data Science and AI can add value to the businesses by empowering management to make better/data driven decisions. It can also help the companies in directing actions based on trends, identifying opportunities, making decisions with quantifiable, data-driven evidence and test these decisions, etc. Let’s look at some examples of how and why companies are using Data Science.
ML usage to Increase Competitiveness
It is hard to be unaware of how machine learning affects enterprises. Machine learning has gained popularity in recent years, and the solutions it offers can be highly advantageous to any business now and in the future. At the moment, enterprises all over the world employ machine learning mostly for:
- Using predictive analysis to improve customer interactions
- Revenue projections and product marketing
- Simplified data management
- Improved selling models
- Awareness of fraud and cybersecurity
However, other companies have advanced machine learning and are now using it in highly inventive ways. For instance, Pinterest used machine learning and data science to build its whole content discovery system. This technology aids the business in anticipating customer preferences and improves the accuracy of search results.
Business process optimization using data science
Data science provides businesses with a wide range of options for streamlining key business procedures. For instance, big data analysis has recently become more prevalent in the manufacturing industry. More and more manufacturing facilities are interested in investing in data analytics and IIoT. (Industrial Internet of Things). Real-time tracking systems and sensor technology gather and analyze data that manufacturers can utilize to:
- Eliminate snags in the production process
- Boost the assets' effectiveness
- Keep track of product flaws and quality
- Conduct product testing
Data science assists firms in minimizing production problems that may have an impact on the product's quality, factory logistics, and shipping procedures. Recruitment is a fantastic illustration of how data science can be used in innovative ways to improve business processes beyond manufacturing.
Now, let’s see how FAANG companies are using Data Science and AI to innovate their products and improve customer experience. We have already covered how the work culture of these FAANG companies is in this article.
How is Facebook/Meta using Data Science and AI?
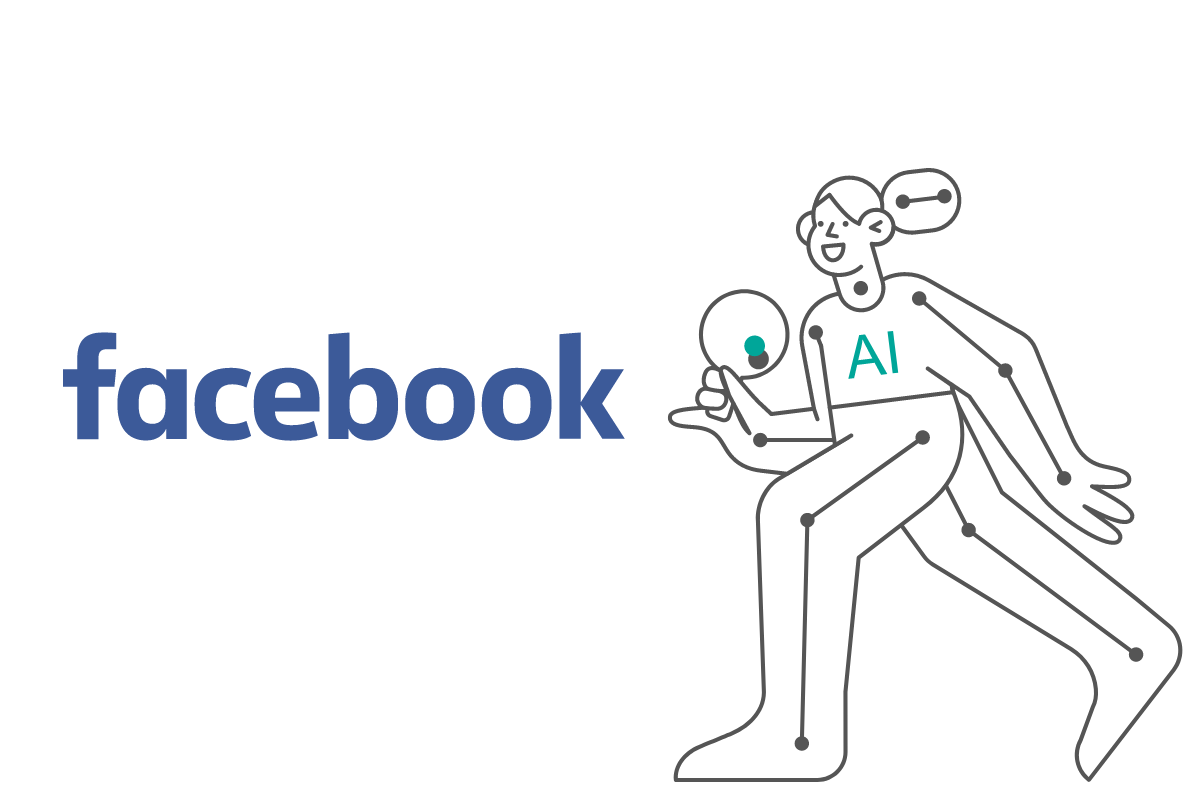
Facebook, now Meta is one of the biggest social media companies in the world. With close to 3 billion monthly active users worldwide, Facebook has captured enormous amounts of data. For Facebook, most of its revenue comes from advertising. So the most important application of data science at Facebook is to decide which advertisements to show to which users.
The company changed the name to Meta since the strategy involved evolving the company into virtual platforms of social technologies where users will be able to immerse themselves in “metaverse”. Facebook has been leveraging data science and AI in every step to make the customer experience better and drive exponential revenue growth. Every 60 seconds on Facebook, 510K comments are posted, 293K status updates and 136K photos are uploaded which is massive. So what does Facebook do with all this data? How do they leverage data science and AI capabilities to make the most of this data? Let's see some examples where Facebook uses Data Science extensively for enhancing customer experience.
Text Analytics
A majority of the data that is available on Facebook is in the form of text; for example, posts and comments unlike that of Instagram which has photos and videos. Facebook has developed an in-house tool called Deep Text which analyzes the text that we shart on post, comments and extract the meaning out of this. This technology is used to identify any abusive posts on Facebook.
Deep Text is a deep learning based text understanding engine that can understand the text by extracting meaning from it at a near-human accuracy level. It is built by leveraging state of the art neural network architectures that can perform word/character level based learning.
Some applications of Deep Text include identifying the sentiment of the post (positive, negative, neutral) or identifying the emotions in the post (sad, happy, angry, threat, etc.). This framework can also be used in identifying the topic; for example whether the post is about Cricket or Football by recognizing the player names from the post.
Topic Data
Facebook has developed Topic Data which leverages data science to help marketers to understand what people are talking about the topics related to their business so that marketers can make their products and marketing relevant to their customers. Earlier to this technology, marketers had to rely on what people are posting online and topics they are talking about but it provided a very limited view. Thus, Facebook developed a framework built by data science to help marketers in building their marketing content more effectively and personalized.
Some examples of how marketers are using Topic Data:
- Inventory manager of a fashion retailer using this data to understand clothing trends of its target audience to decide on which products to stock.
- A company can use this to understand their brand positioning and what the sentiment of their brand is.
- Companies selling a hair de-frizzing product can see demographics data and target relevant customers.
Advertising
Facebook’s main revenue stream is advertising. The company has been using their data very effectively to decide which ads should be shown to which users. On Facebook, we usually see sponsored posts and those are the examples of these targeted advertising practices. When you search for any product on the web and if you are logged in to Facebook, then chances are you will see the product you searched for on your Facebook app as an ad.
Other than the topics discussed above, there are many ways in which Facebook is using data science and AI. Some recent projects that Facebook has undertaken are:
- Detecting Deepfakes - The company recently launched a Deepfake Detection Challenge to build detection models for Deepfake content and to speed up their efforts.
- Language Translation - If you see a Facebook post in another language, Facebook can translate that in real time automatically for you
- Suicide Prevention - Facebook can identify a sentiment of a post and look for signs of trouble in users post and comments and thereby generating alerts and helping people in crisis.
- Image Recognition - Users can easily search through photos without having them to rely on tags or a surrounding test.
How is Amazon using Data Science and AI?
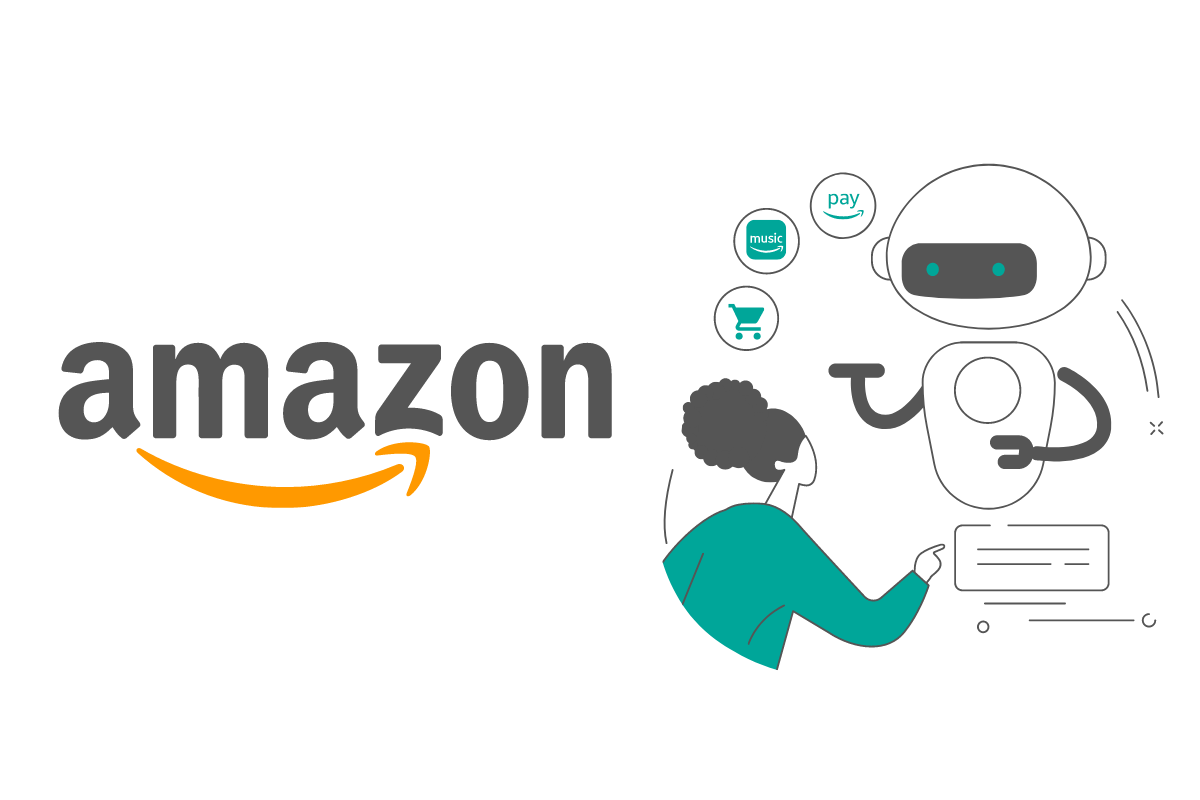
Amazon is one of the largest ecommerce companies in the world. Amazon collects around 1 exabyte of purchase history data from their customers. Apart from online shopping, Amazon provides services like Amazon Web Services, Amazon Pay, Amazon Pantry and many more. With all these services, imagine how much information Amazon has about their customers. They leverage this information to improve the customer experience and drive revenue growth. Amazon collects data about what pages customers visit on their website, what products they are interested in, what category they are interested in and based on all this information, they recommend new products to customers which they are likely to buy.
Amazon is the leader in collecting and processing users' information about how they are spending their money. They use sophisticated data science and machine learning modes for targeted marketing which helps them in increasing customer engagement. Let’s see some of the examples of how Amazon uses Data Science and AI.
Recommendation Engine
With developments in AI, Amazon began working on building a state of the art recommendation engine which would be able to analyze the customer’s behavior on the website and thereby accurately predict what the customer might be interested in the future. The recommendation engine collects a lot of data about the products and the users and then forms relations and dependencies between them.
User-Product relationship occurs when some users with a specific set of characteristics have a similar preference in some products and they buy them often. Example includes Game of Thrones fans buying GoT merchandise and related items. Product-Product relationship occurs when few products on the website are similar in appearance and specifications. For example, if you search for a water bottle and are interested in bottles only for the gym, then all the similar items would be placed together. User-User relationship occurs when a certain set of customers have very similar taste or preference for certain products. For example, tenagers massively buying merchandise from their favorite Youtuber.
Alexa
Amazon offers virtual assistants such as Alexa or Echo/ Echo Show. This product is widely used by the customers to get basic information such as setting reminders, checking weather, checking latest news, etc.
When the users speak with Alexa, the recordings are uploaded to Amazon's servers as voice files and these files are used to train the machine learning algorithms and help make Alexa experience better. Thus, Amazon is continuously collecting data from its users and they use advanced tools in AI to understand what the user is saying. Some customers might not be happy in sharing this voice data and Amazon provides a way to delete these data from their servers.
Pricing Optimization
Amazon changes prices on its products every 10 mins which is close to 2.5m changes in a day across all its products. Amazon has algorithms in place which assess a person’s willingness to buy a specific product. The aim is to set the price of a product in such a way that the customer is likely to buy that product, which is known as dynamic pricing. The changes in the prices occur depending on the user’s activity on the website, competitor’s pricing, product availability, profit margin and many more.
Other than the topics discussed above, there are many ways in which Amazon uses Data Science and AI. One of the latest is using Alexa - Enabled Voice Shopping. This feature uses voice commands as input data and performs the purchasing flows based on the commands. It allows the users to find and purchase products and walk through the checkout flow with voice prompts instead of clicking or tapping on your phone/Echo screen. The goal of this feature is to provide a seamless customer experience for ordering a product and Data Science and AI is at the center of these products.
How is Apple using Data Science and AI?
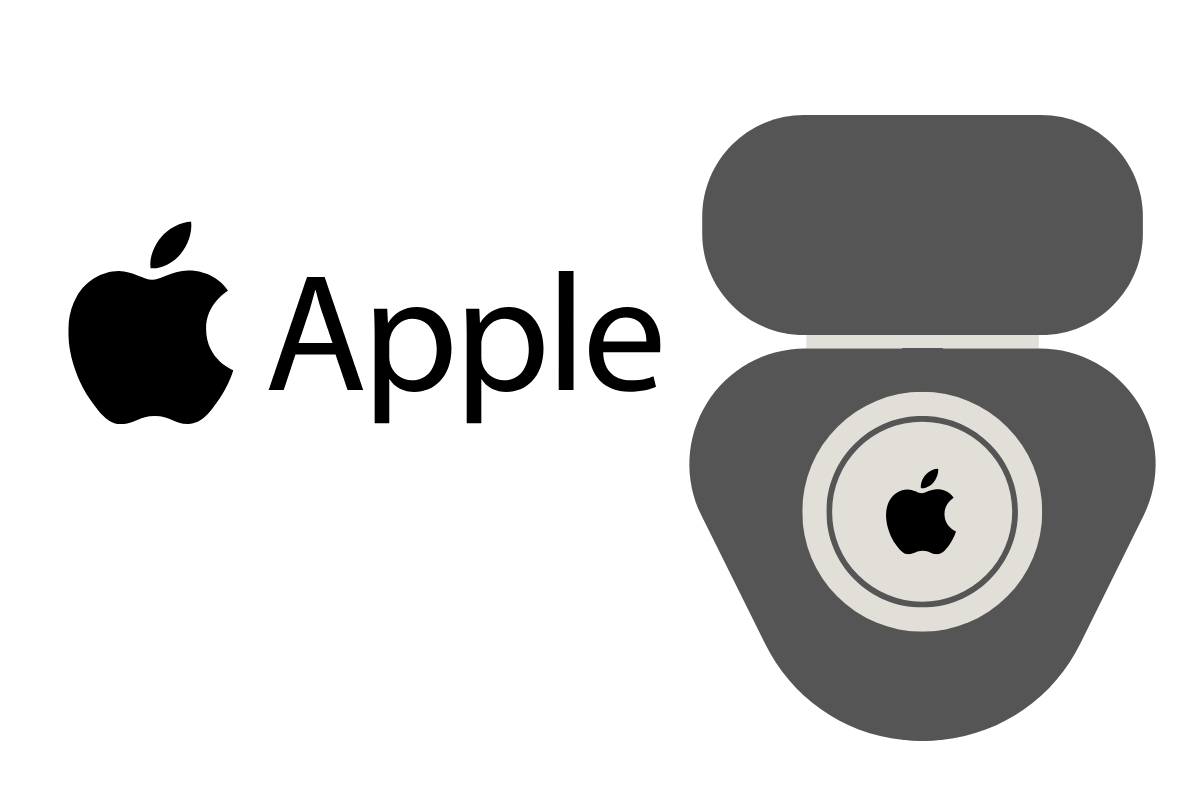
Apple, formerly known as Apple Computers Inc. is a global technology corporation specializing in designing, developing and selling consumer electronics such as Mac, Iphone, Ipad, Airpods, etc. Apple uses AI and Data Science widely to innovate products and build better customer experiences. Let’s discuss some of the AI applications that Apple uses:
Siri
Similar to Alexa, Siri is Apple’s AI-enabled assistant that is available across Apple devices such as Mac, Ipad, Iphone, Airpods, etc. which aims to help the users quickly navigate through the required tasks and perform them quickly without touching the screen. Examples such as setting alarms, reminders, calling someone, weather updates, news, etc.
As a virtual assistant, Siri is built on large-scale ML algorithms that combine speech recognition along with text mining and natural language processing. Firstly, Siri uses speech recognition to translate the human text into textual format and natural language processing is used to identify the meaning of the sentence and prepare the next best response for the user’s task.
Apple Watch Sleep Tracking
Apple has many ways to collect data regarding their customers. One of the most recent ones is the use of Apple Watch where Apple can track user’s activity throughout the day. Apple has partnered with IBM to apply digital information for health management. Using this technology, customers can monitor their health and lifestyle throughout the day and thereby make improvements to it.
Apple Watch also tracks the sleeping patterns and provides data to their customers regarding their deep sleep and light sleep. Based on this data, Apple Watch reminds their users about their sleeping times and how to improve it. These notifications/reminders from the Apple watch are a result of sophisticated machine learning models in the backend.
Apple HomePod
Another AI-enabled technology Apple uses is in HomePod, a speaker powered by Siri that can do more tasks. “With multiple HomePod mini speakers placed around the house, you can have a connected sound system for your whole home. Ask Siri to play one song everywhere or, just as easily, a different song in each room,” (from Keynote speech). Additionally, the speaker can also act as a HomeHub, connecting to Apple’s HomeKit that can be accessed through the iPhone.
Apart from the projects discussed above, Apple uses Data Science and AI in everything they do, especially in innovating their products and technologies. Apple is always ahead in the game of technology and they do this by using big data extensively. The big data technologies in Apple are used to build innovative products such as Siri, Homepod, Apple’s Digital Car Key (partnered with BMW series 5), etc. To conclude, we can say that Apple is utilizing technological advances to improve its user experience by using Artificial Intelligence and Data Science methodologies.
How is Netflix using Data Science and AI?
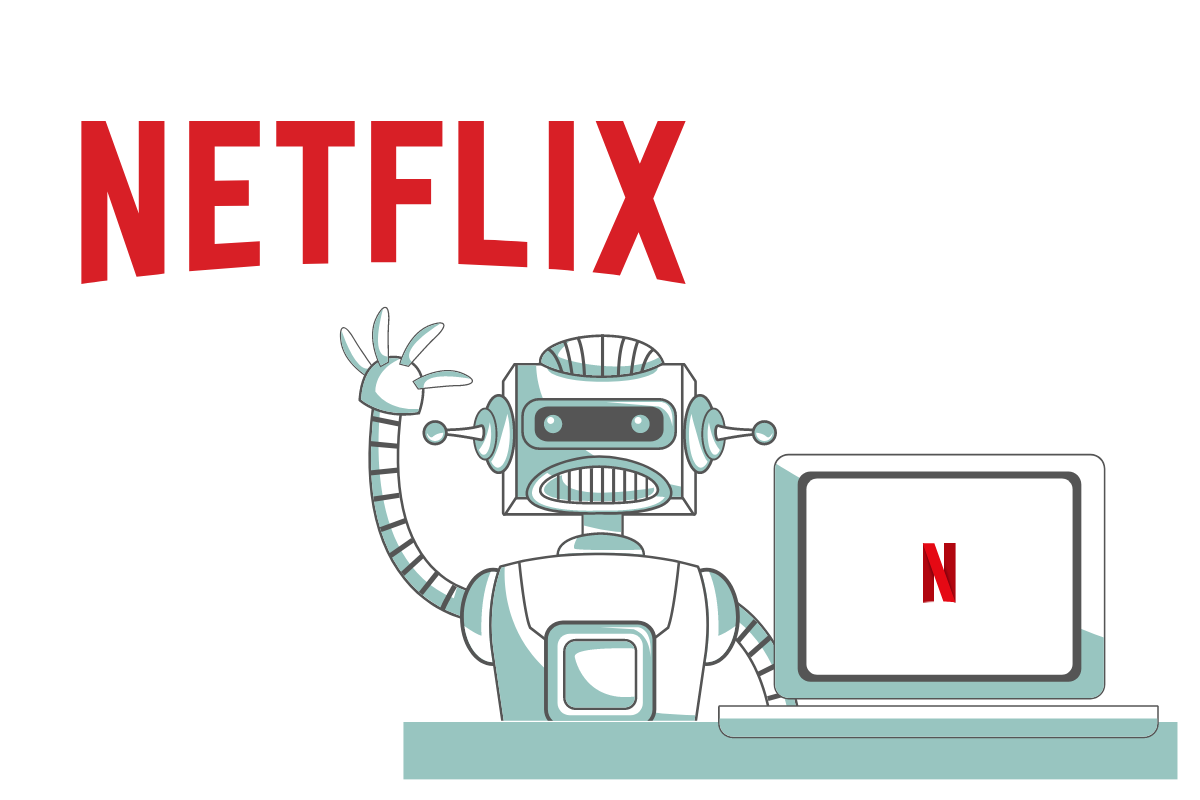
Netflix, originally a DVD-rental service, boomed into one of the biggest video streaming platforms with many options for movies and web series. Netflix generated $24.9 billion revenue in 2021, 23% increase as compared to 2020. In 2022, Netflix has 222 million subscribers worldwide. Netflix has reigned as the number 1 over the top (OTT) streaming platform since its launch in 2007. So what’s the secret of such huge success? It is all about using Data and Analytics to make enhancements in their products and improve customer experience. With the use of data extensively, Netflix can provide users with personalized movie and TV shows recommendations, optimize production planning, predict the popularity of original content, personalize marketing content such as trailers/thumbnail images and help the stakeholders in decision making. Data is at the center of everything that Netflix does. Let’s see some of Data Science applications Netflix uses:
Recommendation Engine
Netflix has built a near-real time recommendation system for their customers by using the huge amount of data they have. Netflix captures information about each user and ranks those users based on what type of content they watch, what they search, what they add to watch-list, etc. This type of data becomes part of Big Data and all this information is stored in the databases which is then used by machine learning algorithms to build a pattern indicating the viewer’s taste. This pattern may match with another user or may not match with anyone since each user might have a unique taste. Based on these ratings to each customer, the recommendation system provides TV shows or movies that the user is likely to watch and enjoy. Thus, they use Data Science extensively to recommend new shows to their end users thereby improving customer experience significantly.
Other than the user behavior on the web-application, Netflix captures data like viewing day, time, location, type of device used, etc. It also captures data around search key-words that a user uses to find a movie/show. Using this data, Netflix is not only able to provide suggestions of the next shows/movies to watch but also arrange the selections into rows based on an individual’s viewing preferences. For example, Netflix will position the program that you are most likely to watch in the top left corner.
Production Planning & Content Development Analytics
At Netflix, Data Science and AI is not only used to understand user behavior for recommendation systems, but it’s also used in production planning and content development activities. When the creators come up with an idea about a movie or the show, data plays an integral part in making any decisions.
Based on the content developed historically and its performance over time, a lot of data is crunched to find insights around what went well and what can be improved. Data around how viewers perceived the previous content is really helpful in predicting the likeability of the new content. For example, Netflix’s executive knew that Umbrella Academy is going to be a hit because it checks certain parameters. It's a series which shows protagonists growth right from childhood to adulthood, features actor Elliot Page and it’s a comic action adventure where all these parameters have been successful in the past.
Also, data is widely used to find out different shoot locations, timings and day of the shoot. Simple prediction models can save them a significant amount of time and effort in planning and reducing the expenses.
Personalized Artwork and Imagery Selection
Netflix knows that imagery plays a very important role in how viewers perceive movies or TV shows to watch. The main objective of the content platform team is to surface those aspects of the story that might be intriguing for the users and increase the chances for that user to watch the tv show or movie. This imagery for a show/movie is purely backed by data science and it is personalized for all the users. Netflix uses Artwork Visual Analysis (AVA), which is a collection of tools and machine learning algorithms that extract relevant imagery from the videos to surface as thumbnails for the customers. Netflix has many tv shows and typically each TV show has around 10 episodes in each season on average which is close to 9 million frames. To select a frame from such a large video manually, which will catch audience attention is tedious and thus, Netflix has developed AVA which does this work automatically. More information on different algorithms used in AVA can be found on their tech blog.
How is Google using Data Science and AI?
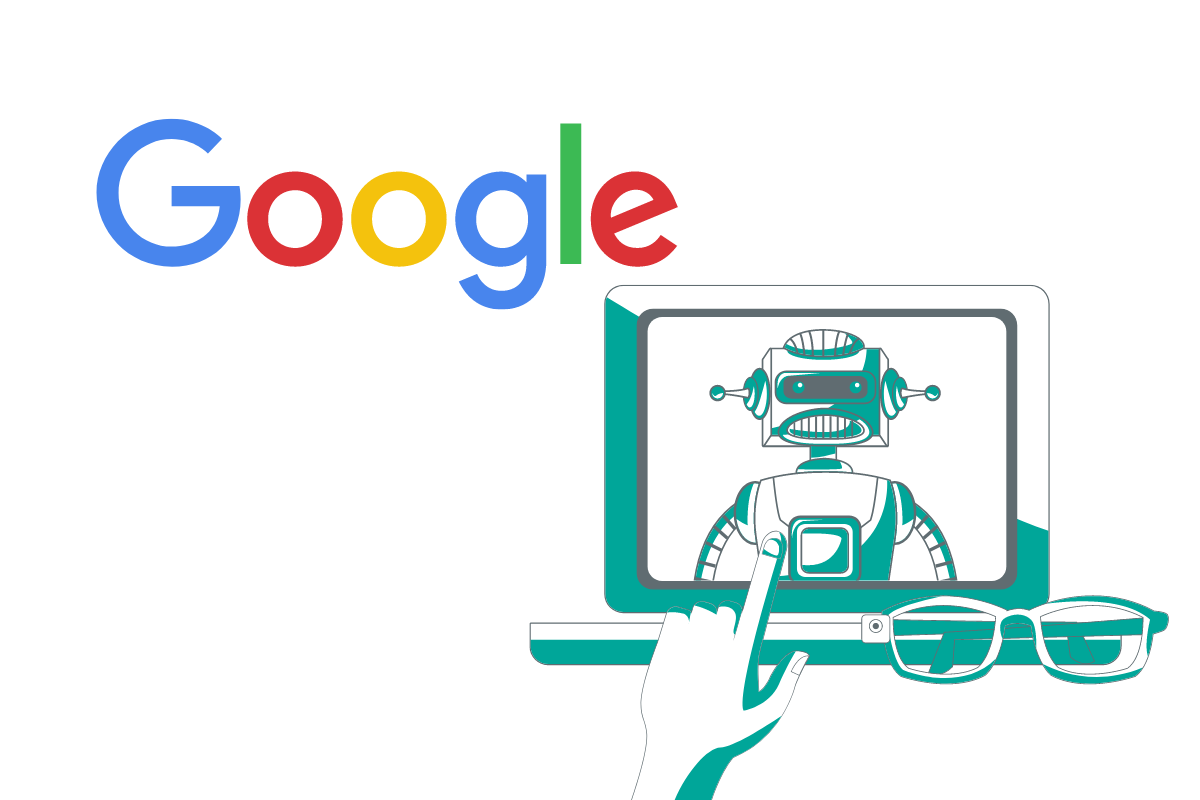
Google is a technology giant which uses Data Science and AI extensively. It is a multinational internet company that provides digital products and services such as online search and advertising, cloud computing and software, etc. Google has a wide range of products and services available and all of them are backed heavily by data science. Google has also acquired Youtube recently which adds on to the list of services that the company offers. Products such as Google Search, Google Photos, Google Drive, G-Suite, Gmail, Voice Search, Reverse Image Search, Maps, speech recognition, Translate and many more. All these products use Data Science to help improve the customer experience and drive revenue growth for the company.
Google Translate
Google Translate is a simple online tool that can be used to translate any text from one language to another language. Earlier in 2006, Google used Statistical Machine Translation for this app but has made great progress in using AI for instant real time translation of text. The latest machine learning algorithms Google Translate uses, provides translation in 109 different languages and it has boosted the quality and reliability of these translations. Google has made significant improvements in the field of Natural Language Processing which enabled them to have a high accuracy rate in Google Translate.
Google Ads
Google Ads, formerly known as AdWords is a part of Google’s marketing suite of tools. Google Ads gives full control to the businesses and users to advertise their products online and profile the users based on their searches. This data is used by Google to target the right advert to the right users which is a main idea behind Google Ads. Google uses state of the art machine learning algorithms that rank thousands of keywords based on several metrics, which are then used to pick the right ad to show to the users.
Gmail
Gmail has been used by a lot of customers as their primary email service and Google has implemented many smart features to it. One of the latest smart features is called a smart reply. This feature reads the email, extract the meaning of that email and provides the user with possible responses so that the users don’t have to type a lot. Also, Google uses machine learning algorithms to identify and categorize emails as Spam or not-spam. Another Data Science backed feature in Gmail is automatic categorization of emails into Promotions, Social, Updates, Priority, etc.
Apart from the products discussed above, Google uses Machine Learning and AI to build smart products. Google is leading in the technology space due to heavy investments in research in advanced computer science and artificial intelligence.
Conclusion
Any organization that uses its data effectively can benefit from data science. Data science is beneficial to any business in any industry, from statistics and insights throughout workflows and employing new applicants to assisting senior employees in making more informed decisions.
If your aim is to work for either of the above data science companies, it’s very important to develop skills needed for a Data Scientist / Data Engineers, etc. depending on your preference. With StrataScratch, you can tackle coding as well as non-coding questions and would be able to be a part of a community of like minded people. You can communicate and collaborate with other aspiring Data Engineers and work towards achieving your dream job. We have more than 400 real life SQL questions on the platform ranging from beginner to advanced level. We highly recommend you joining the community of over 20K learners and be interview ready. All the best!
Share