314 Resources For Data Science Fundamentals
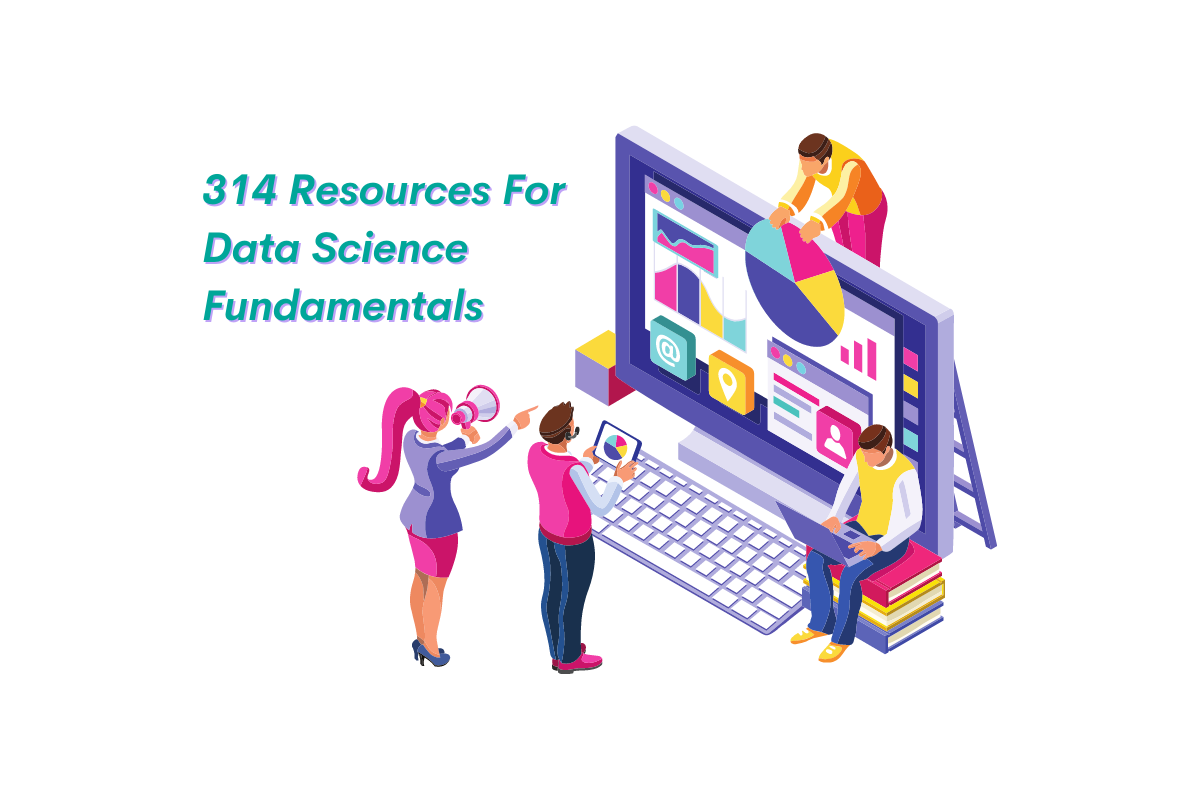
Categories:
- Written by:
Abhishek Ramesh
A list of best resources for data science fundamentals.
This is a fundamentals guide to data science interviews. In this guide, common topics from coding and non-coding questions asked during data science interviews are covered!
The 7 categories covered are:
- Python
- SQL
- Probability
- Statistics
- Modeling
- Business Case
- Product
The common topics asked are classified under their relevant subject. For example, Regression would be classified under the Statistics category. These common topics include YouTube videos or articles explaining the topic. Most topics also include interview questions from companies such as Google and Uber for practice.
While this guide provides common topics asked during data science interviews, this fundamentals guide is meant as a base page. You should further research on the topics mentioned.
- For coding questions, it is all about practice. Practicing and understanding your own and other people’s code. Some people may have a solution that is more optimized than yours, which is why building a community is important. StrataScratch shows how other people have solved a question you attempted, where you can also learn more about how to better your solution!
- For non-coding questions, you need to understand each step of applying a concept. The understanding of mathematical derivations behind a concept is important to truly answer any question about the relevant concept. Once you understand the math behind a concept, you need to learn how to effectively program these concepts. This comes back to practice and learning from others. It is important to understand the mathematical derivation of concepts. While only a handful of interview questions will ask mathematical knowledge behind certain topics, such as logistic regression, it is good to know when implementing complex models during your job as a future data scientist!
There are specific topics that are only asked by a handful of companies. To get a better idea of the type of potential topics companies may ask, you should go through the job requirements. However, some companies do not write their Data Science job descriptions when hiring. They may just copy descriptions from Glassdoor or might ask for someone who knows every single programming language and statistical models out there. When you notice job descriptions similar to this, you should be good with going through the topics mentioned in this guide.
It must be noted that not all topics include a practice question. Even if a topic does not include a practice question, you should understand the concept, since this may be asked during your interview or future data science job!
If you do not understand a topic, check out the StrataScratch blogs! There are multiple guides written to help interviewers prepare from SQL Window Functions to Collinearity. If there are topics that are not explained in the StrataScratch blogs, visit other websites to learn more about the topic! Take notes about these topics and go through more practice questions! It is recommended to have a document of topics you don’t fully understand or easily forget.
For example, if you easily forget how the ranking() function works in SQL take screenshots and notes to explain the concept.
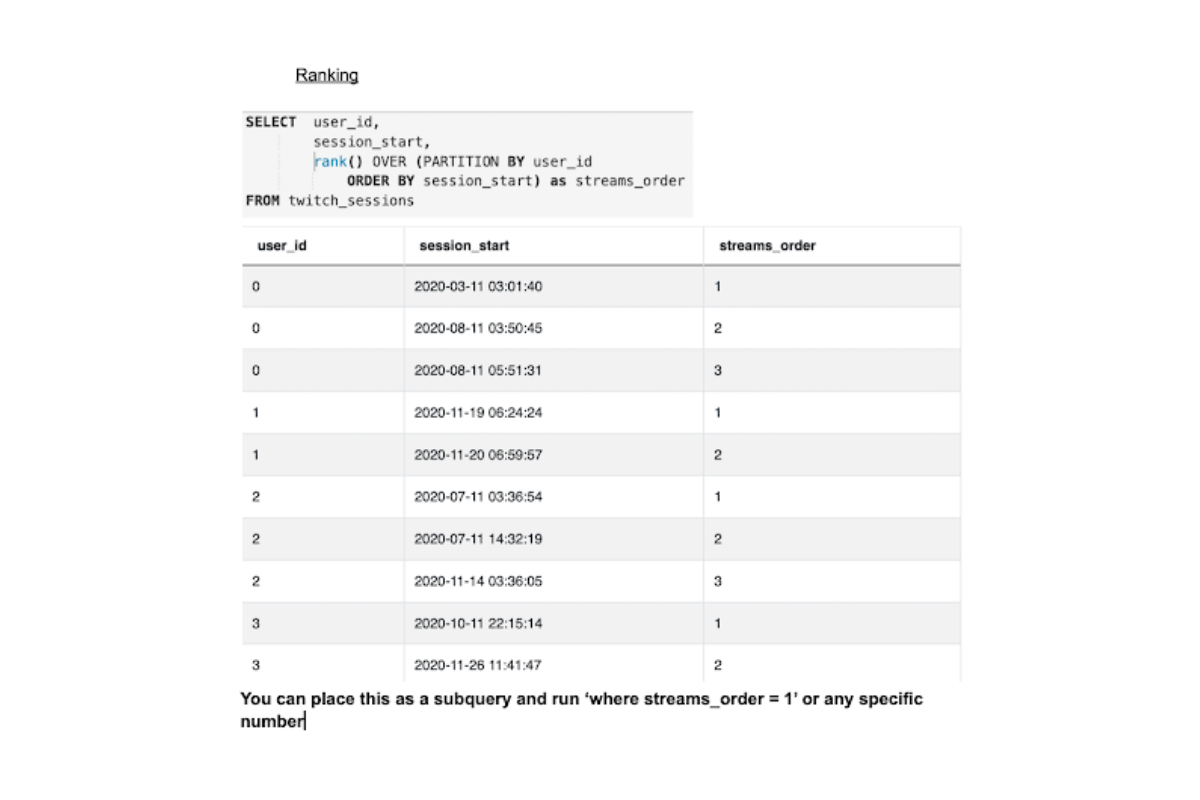
Do keep in mind that medium/hard questions on the StrataScratch database will contain multiple concepts, since during actual data science interviews you will need to know a variety of functions/topics.
When a question asks you to explain a certain concept to a specific target audience, think in the eyes of the target audience when answering these types of questions. Remember to not use technical jargon that would confuse the audience, especially a non-technical audience. For example, this question asks how to explain regression to an 8 year old. Obviously an 8 year old would not understand MSE values or even a simple y=mx+b equation, so explain using everyday jargon.
Coding Questions
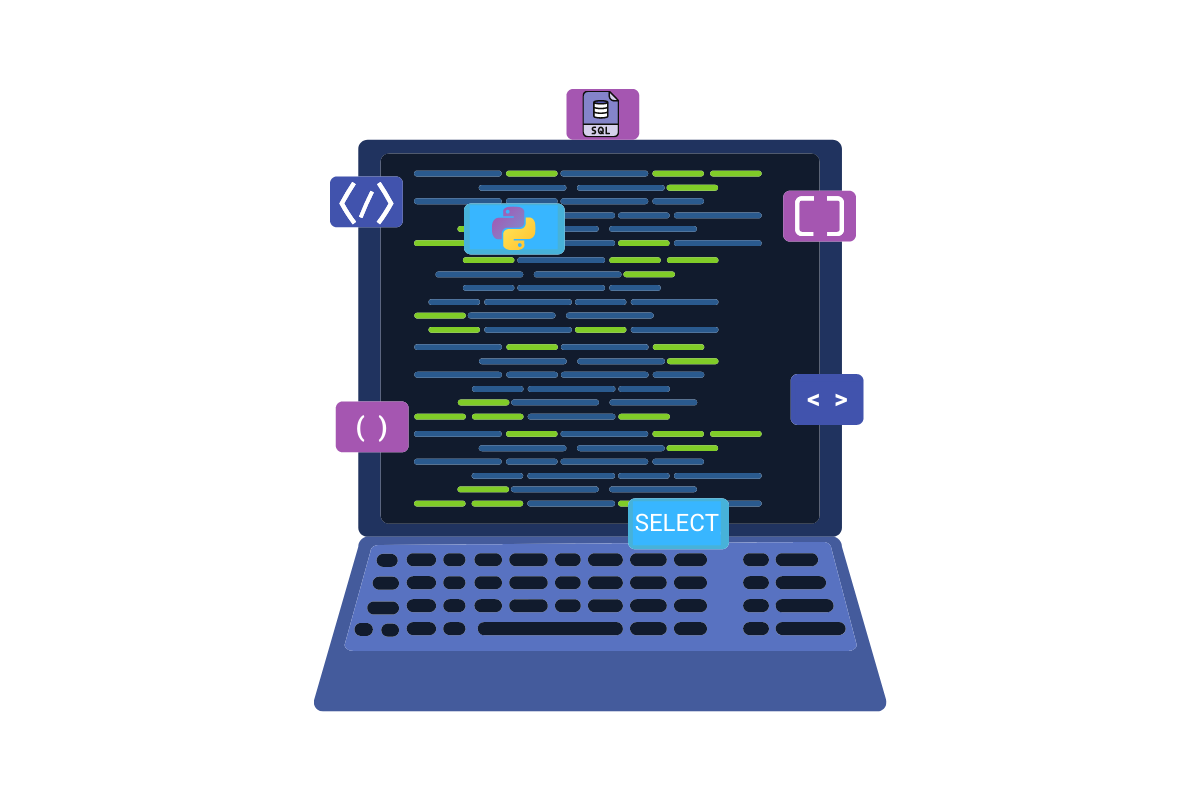
Python
Everyone who knows how to code nowadays has heard of Python. There is a reason Python is extremely popular among Data Analysts and Data Scientists. It has a variety of libraries and the ability to process data quickly. NumPy and Pandas are some of the most commonly used libraries for data analysis.
- Understanding NumPy / Pandas
- Comparison Operators / Logical Operators / Mathematical Functions
- Flow Control Functions
- Conditional Expressions
- Filtering by columns/rows
- Unique values - nunique() / drop duplicates
- Null values
- Casting data types
- Rounding values
- Sorting
- DataFrame Formatting
- Ranking Methods
- Dict Methods
- Array Operations
- Learning
- Practice
- String Functions
- List Methods
- Lambda function
- Class methods/Set Methods
SQL
Even though Python and Pandas has the ability to process databases, Pandas takes much longer and sometimes can not handle larger databases. In these cases, Structured Query Language (SQL) is highly preferred. SQL has simpler code while having the ability to filter and restructure databases through queries.
In the following sections, there will be multiple links to learning and practicing specific concepts, a free one-stop for SQL tutorial is https://mode.com/sql-tutorial. However, it is recommended to go through YouTube SQL tutorials as well, especially if it is your first time learning, so you can learn tips and tricks when coding.
Functions
- Where / Sorting / Having
- Limit / Offset
- Date Time Functions
- Distinct Clause
- Aggregate Functions (Group By, Case)
- Combining (Joins / Unions)
- Subquery Expressions
- Window Functions (Partition by, Rank, Ntile, Lag/Lead, Common Table Expression)
- Learning
- SQL Window Functions on Data Science Interviews in 2021 | Asked By Airbnb, Netflix, Twitter, Uber
- SQL Coding Interview Question Using A Window Function (PARTITION BY) | Data Science Interviews
- Multiple Solutions to Data Scientist Interview Question From Amazon [Rolling Average]
- What a Moving Average Is and How to Compute it in SQL
- Common Table Expressions – The Ultimate Guide
- Practice
- Learning
- Pattern Matching / Text Searching
- Array Functions
Non-Coding Questions
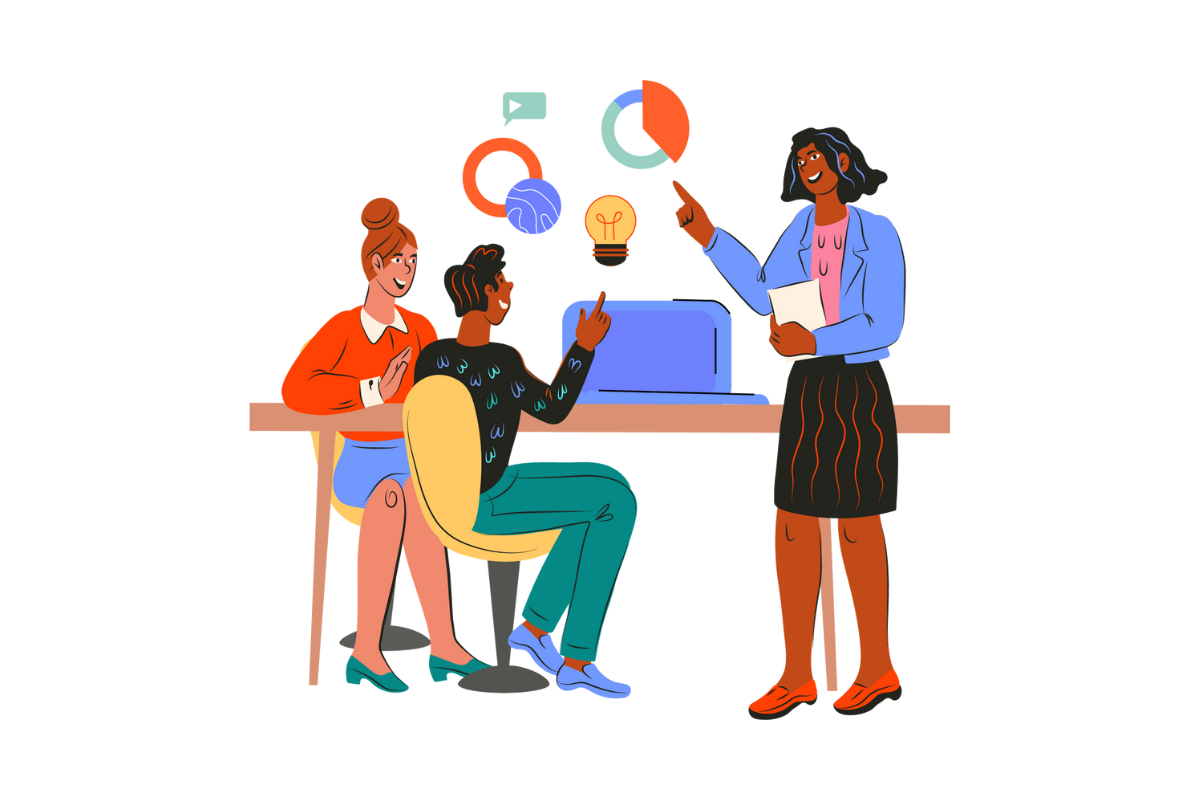
Probability
Understanding mathematical concepts is an important part of being a successful data scientist. Probability provides an important foundation for concepts such as Bayes Theorem and distributions.
- Axioms of Probability
- Learning
- Permutations/Combinations
- Multiplication Rule
- Conditional Probability
- Independent Events
- Bayes Theorem
- Learning
- Practice
- Different distributions
- Probability Density Function and Cumulative Density Function
- Normal Distribution (aka Gaussian Distribution)
- Uniform Distribution
- t Distribution (aka Student’s t distribution)
- F Distribution
- Chi-Squared Distribution
- Exponential Distribution
- Lognormal Distribution
- Learning
- Lognormal Distribution
Discrete
- Lognormal Distribution
- Learning
- Binomial Distribution
- Poisson Distribution
- Series: Geometric - Hypergeometric - Arithmetic - Summation to Infinity
- Expected Value
- Binomial Distribution - Negative Binomial
Statistics
Data Science can be summed up as Computational Statistics. From predicting what shows are recommended to you on Netflix (Collaborative filtering) to predicting the demand of iPhones next year (Regression), statistics is the basis of Data Scientists.
- Intro to Stats (Mean, Median, Mode, Range, Standard Deviation, Graphs)
- Boxplot - IQR
- Learning
- Practice
- Variance → ANOVA
- Z-test --- T-test
- Learning
- Z-statistics vs. T-statistics | Inferential statistics | Probability and Statistics | Khan Academy
- https://www.ztable.net/wp-content/uploads/2018/11/negativeztable.png
- http://www.ttable.org/uploads/2/1/7/9/21795380/published/9754276.png
- Hypothesis Testing Problems Z Test & T Statistics One & Two Tailed Tests 2
- Practice
- Learning
- Central Limit Theorem
- Confidence Interval
- Hypothesis testing -- P-Value
- Confusion matrix (Sensitivity and specificity)
- A/B testing
- Polar Coordinates
- Correlation coefficient (aka Pearson's correlation coefficient)
- Bias-Variance Tradeoff
- Error Predictions (MSE, RMSE, MAE, R^2)
- Learning
- Practice
- Regression
- Learning
- OLS - Introduction to residuals and least squares regression
- Ridge - Regularization Part 1: Ridge (L2) Regression
- Lasso - Regularization Part 2: Lasso (L1) Regression
- Elastic-Net - Regularization Part 3: Elastic Net Regression
- Logistic Regression - StatQuest: Logistic Regression
- Regularization: Ridge, Lasso and Elastic Net
- Logistic vs Bayesian Logistic
- Practice
- Learning
- F-statistic
Modeling
Modeling is the application of statistical concepts and frameworks in everyday scenarios. Before attempting these questions make sure you have a thorough understanding of the concepts in the Statistics section.
Structuring Data
- Overfitting/Underfitting
- Cleaning up data
- One-hot/Label/Ordinal encoding
- Missing values
- Learning - 7 Ways to Handle Missing Values in Machine Learning
- Practice - Scikit-learn Models Missing Values
- Diagnostic Tests (Outliers, Collinearity, Normality, Autocorrelation, Linearity, Homoscedasticity/Heteroscedasticity, Stochastic)
- Outliers
- Box-plot - How To Make Box and Whisker Plots
- Grubbs Test - Grubbs Test (example)
- Randomness
- Chi-Squared - Chi Square Test
- Collinearity
- Normality
- Shapiro-Wilk - Shapiro-Wilk test
- Kolmogorow-Smirnov test - 10: Kolmogorov-Smirnov test
- What is the difference between the Shapiro-Wilk test of normality and the Kolmogorov-Smirnov test of normality?
- Autocorrelation
- Durbin-Watson test - Serial correlation - The Durbin-Watson test
- Homoscedasticity / Heteroskedasticity
- Homoscedasticity - Bartlett's test
- Heteroskedasticity - Breusch–Pagan test - The Breusch Pagan test for heteroscedasticity
Note: While these are common tests that are usually used in Data Science interviews, there are a lot more tests and more types of diagnostic tests than mentioned above.
- Outliers
- Transformations (bptest, box-cox transformation, SIFT)
- SIFT
- Learning - Introduction to SIFT( Scale Invariant Feature Transform)
- Practice - SIFT
- Box-Cox transformation
- Log transformation
- SIFT
- Testing and Training Data Split + Cross-validation
Modeling Data
- Supervised vs Unsupervised Learning
- Feature Extraction
- PCA
- Factor Analysis
- Linear/Quadratic Discriminant Analysis
- Feature Selection
- AIC
- BIC
- Mallow’s Cp
- Learning - What is Mallows’ Cp? (Defintion & Example)
- Adjusted R^2
- Stepwise Regression/Forward Selection/Backward Elimination
- Regression -- [Explained in statistics]
- Forecasting
- Learning - An overview of time series forecasting models
- Practice - Time Series Forecasting Techniques
- Ensembles
- Boosting (XGboost)
- Random Forests
- Decision Trees
- K-means clustering
- Learning
- Practice
- Naive Bayes Classifier
Neural Networks
- SVM
- Gradient Descent + SGD
- Neural Networks (CNN/RNN)
Not all modeling questions will explicitly mention a statistical concept to use as part of your answer. Interviewers test your understanding of the questions and what techniques you will use to solve the question. Remember that interviewers don’t always look for the most accurate solution, but want to see you have a solid understanding about the question and how to go about solving it. Remember before attempting these questions during an actual interview, ask clarifying questions such as ambiguous terminology to the interviewer.
Generalized
The following questions test your understanding of when to use which statistical analysis and your overall approach to an everyday problem. For example, a modeling question asked by Amazon was to predict whether a customer will buy something today or not based on their information. Definitely practice these before your interviews!
- Linear Regression Optimization
- Active User Probability
- Credit Risk Scoring Model
- Model Parameters
- Better Algorithm
- Rate a Movie
- Data Set with 500 Features
- Customer Prediction
- Purchase Intent
- Imbalanced Data Issues
- Credit Card Fraud Model
- Perfect Classification Model
- NFL Games
- Flight Delay Regression
- Users in Meaningful Segments
- Categorical Dependent Variable
- Data Quality Issue
Business Case
Business case questions are a tricker type of questions. These questions can not be split into basic topics to learn. These questions are split into 3 topics: applied data, sizing, theory testing. These questions mainly test your understanding of the company’s products, economy and business competitions.
These are the types of questions to practice multiple times. If you want to get even better, research the company’s products before the interview, so you can show the company you’re interested in them.
Fortunately, we have written a guide to solving data science business case questions to learn more about how to improve on answering business questions.
Product
Product questions, similar to business case questions, can not be split into topics to learn from scratch. These questions are split into 3 topics: metric related problems, measuring impact of a new product/feature, and designing products. These are questions to practice repeatedly. Fortunately, we have written an ultimate guide to solving data science product questions. To learn more about how to improve on solving product questions, check out the ultimate guide to product data science interview questions.
Share